Foundation Model and Reinforcement Learning
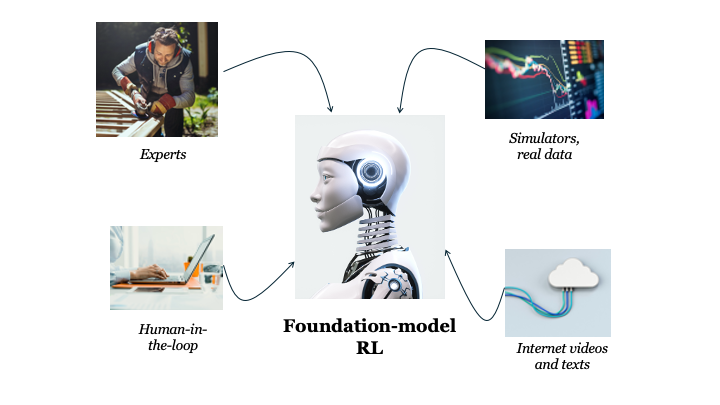
One of the main challenges in control is generalization to diverse and unseen tasks. Conventional control methods and modern Reinforcement Learning (RL) approaches have focused on task-specific solutions or a tabula rasa approach. These methods learn to solve one task from scratch without incorporating broad knowledge from other datasets. How can decision-making algorithms 1) adapt to modalities not covered in the datasets, 2) generalize to unseen tasks, and 3) adapt to a specific task? The intersection of foundation models and RL holds tremendous promise for creating powerful control systems that can switch and adapt to a diverse range of tasks.
A foundation model is trained on broad datasets, enabling it to be applied across a wide range of use cases. Foundation models facilitate the creation of larger datasets for learning multitask and generalist RL agents by serving as generative models of behavior and generative models of the system.
Contact
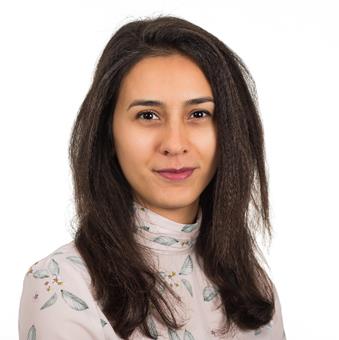
Farnaz Adib Yaghmaie
Assistant Professor
Linköping University