Robust Decision-Making Under Uncertainty and Model Errors
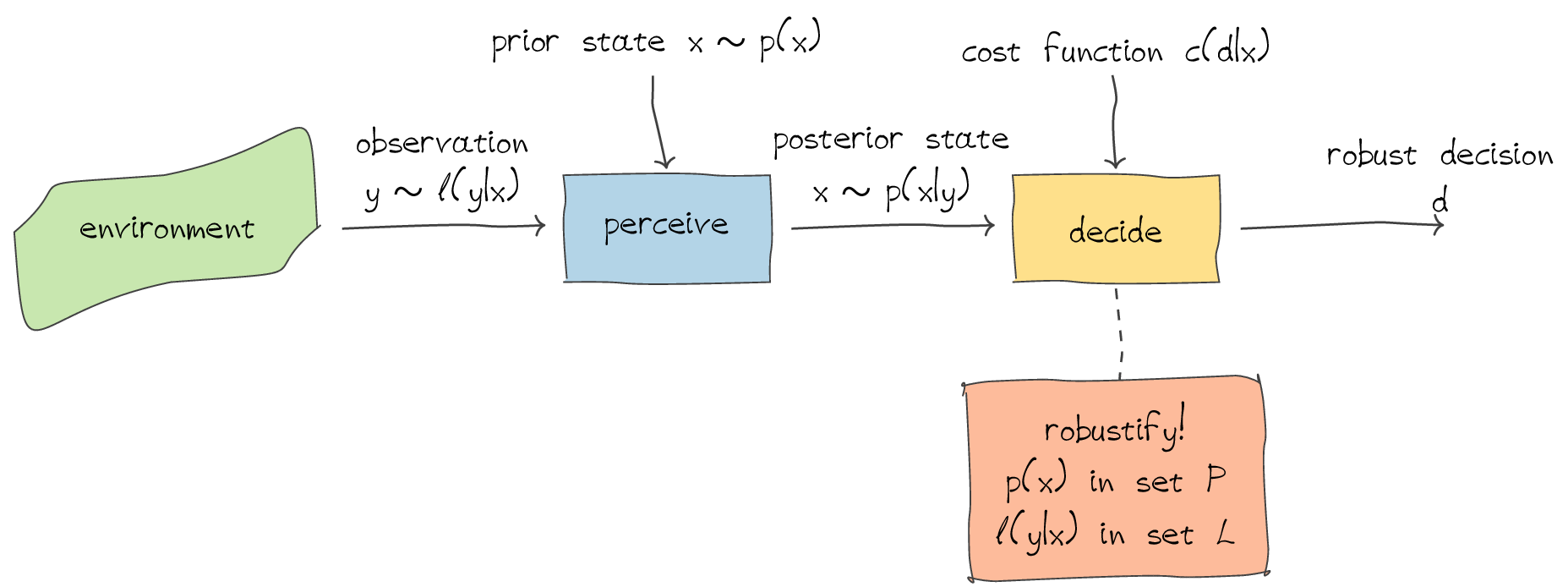
Autonomous decision-making under uncertainty is a challenging task. One main reason is that autonomous systems deploying decision-making algorithms must operate across a wide range of scenarios, requiring the use of generic mathematical models. Such models, however, cannot be tailored and optimized for all use cases simultaneously, meaning that decision-making problems will inherently include model errors. This project studies decision-making under uncertainty, focusing on both state estimation uncertainties and model errors. A key performance indicator is robustness with respect to model errors. Initially, we will investigate the following ideas:
- Robust Bayesian analysis. A framework for assessing the robustness of Bayesian decision problems concerning uncertainties in priors, likelihoods, and cost functions. For instance, the decision sensitivity can be examined by varying the prior within some set of prior distributions.
- Human-inspired decision making. Humans can make robust decisions in highly complex situations with incomplete information. Drawing inspiration from human decision-making processes, we consider prospect theory and regret theory as two compelling frameworks for human-like decision-making.
Contact
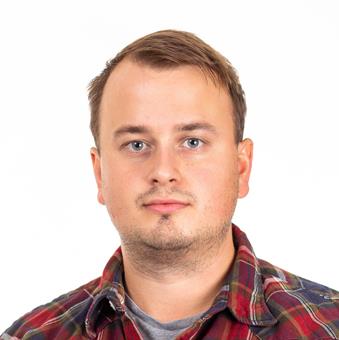
Robin Forsling
Industrial Postdoc
Linköping University